Unmasking The Power Of Data Protection: A Guide To Data Masking
3 Mins Read
Published on: 07 September 2023
Last Updated on: 18 March 2025
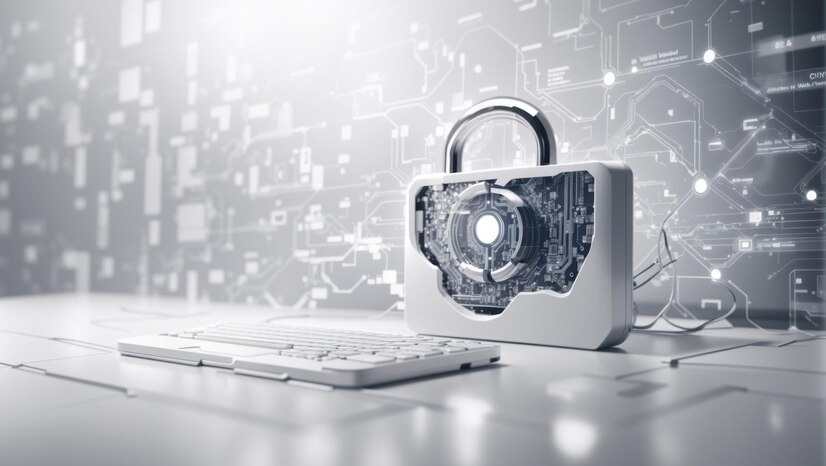
toc impalement
In the digital age, data is often touted as the new oil, powering businesses and driving innovation.
However, with great data comes great responsibility, especially when it comes to protecting sensitive information. Data masking, a critical component of data security, plays a pivotal role in safeguarding sensitive data while still allowing organizations to utilize it for various purposes.
In this article, we’ll delve into the world of data masking, and its importance, and explore the emerging concept of synthetic data generation as a complement to data security.
The Need For Data Protection
Data has become the lifeblood of modern enterprises, underpinning decision-making, customer service, and numerous other aspects of business operations. Yet, not all data is meant to be freely accessible. Personally identifiable information (PII), financial records, and healthcare data, among others, must be protected against unauthorized access and breaches.
The consequences of data breaches can be dire, encompassing financial losses, regulatory fines, reputational damage, and legal repercussions. As a result, organizations are turning to data protection strategies, with data masking being one of the most effective and versatile methods.
Understanding Data Masking
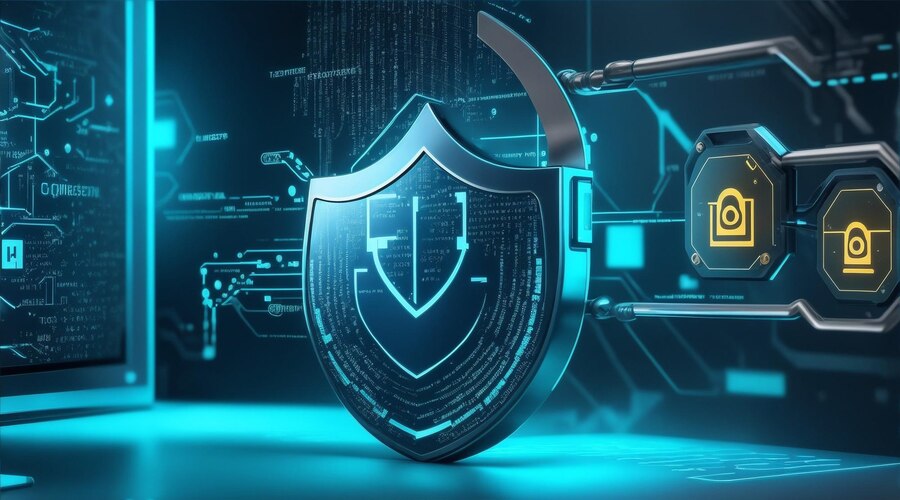
Data masking, also known as data obfuscation or data anonymization, is the process of concealing original data with fictional, yet realistic, information. The primary objective is to make sensitive data unreadable or unusable by unauthorized users while maintaining its usability for legitimate purposes.
How Data Masking Works:
- Data Discovery: The first step involves identifying sensitive data elements within the dataset, such as Social Security numbers, credit card numbers, or email addresses. Additionally, utilizing data discovery tools, businesses can automate the detection of structured and unstructured sensitive data across multiple environments. These tools help ensure compliance, reduce security risks, and provide greater visibility into where critical information is stored and how it is accessed.
- Masking Techniques: There are various data masking techniques available, including substitution (replacing sensitive values with fictional ones), shuffling (randomizing the order of values), and encryption (transforming data using encryption algorithms).
- Preservation of Format: It’s crucial to preserve the format and structure of the original data to ensure that applications and processes relying on this data remain functional.
- Testing and Validation: Before deploying masked data, thorough testing and validation are essential to ensure that the masked data remains consistent, secure, and functional.
Benefits of Data Masking:
- Security: Data masking protects sensitive data from unauthorized access and reduces the risk of data breaches.
- Compliance: It helps organizations comply with data protection regulations like GDPR, HIPAA, and CCPA by ensuring that sensitive data is not exposed.
- Usability: Masked data can still be used for testing, development, and analytics without revealing sensitive information.
- Risk Mitigation: It reduces the risk associated with data exposure during development and testing phases.
Synthetic Data Generation: Enhancing Data Masking
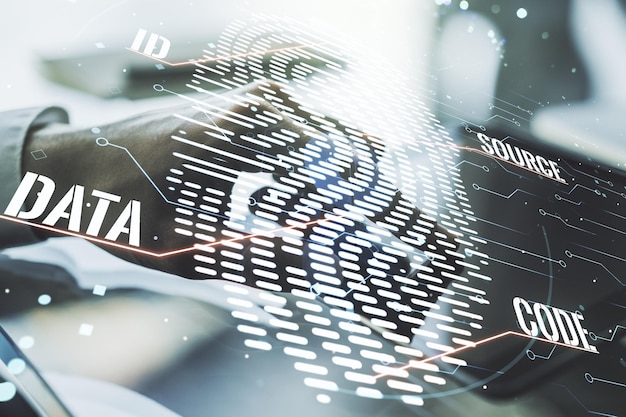
While data masking is a robust technique for protecting sensitive information, it has limitations. In some cases, organizations may require data that closely resembles the original dataset but lacks actual sensitive information. This is where synthetic data generation comes into play.
What Is Synthetic Data?
Synthetic data is artificially generated data that mimics the statistical properties and characteristics of real data without containing any actual sensitive information. It allows organizations to create datasets that are highly similar to the original, making it ideal for various applications like testing, development, and analysis.
- Combining Data Masking and Synthetic Data Generation:
- By combining data masking and synthetic data generation, organizations can maximize data security while retaining data usability. Here’s how it works:
- Mask Sensitive Data: First, sensitive data is masked using appropriate techniques to protect it.
- Generate Synthetic Data: Synthetic data is then created to replace the masked sensitive data, ensuring that the dataset’s structure and statistical properties are maintained.
- Usability and Security: The resulting dataset is both secure and usable, suitable for various purposes without exposing sensitive information.
Conclusion
Data masking and synthetic data generation are powerful tools in the arsenal of data protection and privacy. In a world where data breaches are a constant threat, it is imperative for organizations to adopt these techniques to safeguard sensitive information while still leveraging data for business purposes.
As data continues to be a driving force in the digital landscape, the integration of robust data protection measures like data masking and synthetic data generation will become increasingly vital in securing our digital future.
Read Also:
Comments Are Closed For This Article